Cost Optimization for Logistics Services: A Simulation Approach to Delivery Alternatives
DOI:
https://doi.org/10.12928/si.v22i2.195Keywords:
Logistics, Travelers, Simulation, Cost, Late penaltyAbstract
An essential activity in the delivery of goods by logistics service companies is how to deliver goods to consumers according to the agreed time with minimal costs. A case study was conducted on one of the logistics service companies in Bandung, which has an exciting feature: promising goods to consumers within 24 hours. The interesting thing about this company is that it uses the rest of the luggage of travelers traveling to the destination city by plane. In existing conditions, problems often arise, namely, goods do not reach customers according to the agreed time. This causes losses to the company because it must pay a late penalty. Therefore, the author designed several alternatives to meet freight forwarding in less than 24 hours. This study aims to optimize the cost of shipping goods from various alternatives by considering the delivery time of less than 24 hours. This study uses an experimental method with a system model to conduct simulations. Parameters use primary data from the company and secondary data from websites. The author designed two alternatives to shipping goods if no match was found with the traveler. The first alternative is to use air cargo at Bandung Airport. The second alternative is that if it is predicted that the goods will not reach the customer within 24 hours through Bandung Airport, they will be sent to Soekarno Hatta Airport Jakarta using a truck. A match with the traveler at the airport will be sought. The second alternative is also considered if there is no match with the traveler, then the delivery of goods uses air cargo. The simulation results provide a total cost for alternatives 1 and 2 of IDR 69,779,084.40/month and IDR 107,025,296, respectively, for goods that do not meet the delivery of less than 24 hours for alternative 1, namely nine items/month or 1% of the total shipment and alternative 2, namely 19 goods or 2% of the total delivery. The simulation in this study resulted in choosing the first alternative as the best alternative with the lowest cost.
References
Akinyemi, Y. C. (2023). Air cargo demand in Africa: Application of cointegration and error correction modelling techniques. Journal of Air Transport Management, 109, https://doi.org/10.1016/j.jairtraman.2023.102399.
Banerjee, D., Erera, A. L., Stroh, A. M., & Toriello, A. (2023). Who has access to e-commerce and when? Time-varying service regions in same-day delivery. Transportation Research Part B: Methodological, 170, 148–168, https://doi.org/10.1016/j.trb.2023.02.005.
Benamou, J. D., Froese, B. D., & Oberman, A. M. (2014). Numerical solution of the Optimal Transportation problem using the Monge-Ampere equation. Journal of Computational Physics, 260, 107–126, https://doi.org/10.1016/j.jcp.2013.12.015.
Boysen, N., Briskorn, D., & Rupp, J. (2023). Optimization of two-echelon last-mile delivery via cargo tunnel and a delivery person. Computers and Operations Research, 151, https://doi.org/10.1016/j.cor.2022.106123.
Chen, X., Wang, T., Thomas, B. W., & Ulmer, M. W. (2023). Same-day delivery with fair customer service. European Journal of Operational Research, 308(2), 738–751, https://doi.org/10.1016/j.ejor.2022.12.009.
Girardet, D., & Spinler, S. (2013). Does the aviation Emission Trading System influence the financial evaluation of new airplanes? An assessment of present values and purchase options. Transportation Research Part D: Transport and Environment, 20, 30–39, https://doi.org/10.1016/j.trd.2013.01.002.
Glass, C., Davis, L., & Watkins-Lewis, K. (2022). A visualization and optimization of the impact of a severe weather disruption to an air transportation network. Computers and Industrial Engineering, 168, https://doi.org/10.1016/j.cie.2022.107978.
Harahap, D. A., & Amanah, D. (2018). PERILAKU BELANJA ONLINE DI INDONESIA: STUDI KASUS. Jurnal Riset Manajemen Sains Indonesia (JRMSI) |, 9(2), 2301–8313, https://doi.org/10.21009/JRMSI.
Horng, S. C., & Lin, S. S. (2024). Advanced golden jackal optimization for solving the constrained integer stochastic optimization problems. Mathematics and Computers in Simulation, 217, 188–201, https://doi.org/10.1016/j.matcom.2023.10.021.
Kovacikova, K., Novak, A., Kovacikova, M., & Sedlackova, A. N. (2023). Comparison of selected airports in terms of sustainability. Transportation Research Procedia, 75, 53–59, https://doi.org/10.1016/j.trpro.2023.12.007.
Kumar Kushwaha, D., Sen, G., Aakash, A., & Thomas, S. (2024). Air cargo transportation, loading, and phase-based maintenance service scheduling in demand channel routes. Computers & Industrial Engineering, 110341, https://doi.org/10.1016/j.cie.2024.110341.
Kumar Phanden, R., Sheokand, A., Kumar Goyal, K., Gahlot, P., & Ibrahim Demir, H. (2022). Analisis Penyebab Keterlambatan Pengiriman Barang pada Pos Express Menggunakan Metode Six Sigma. Materials Today: Proceedings, 65, 3266–3272, https://doi.org/10.1016/j.matpr.2022.05.383.
Levashev, A., Pavlova, O., Sokolova, N., & Chelpanova, I. (2022). Principles of Allocation of Special Transportation Analysis Zones. Transportation Research Procedia, 68, 876–883, https://doi.org/10.1016/j.trpro.2023.02.124.
Li, N., Wu, Y., Ye, H., Wang, L., Wang, Q., & Jia, M. (2024). Scheduling optimization of underground mine trackless transportation based on improved estimation of distribution algorithm. Expert Systems with Applications, 245, https://doi.org/10.1016/j.eswa.2023.123025.
Li, Y., Gu, W., Yuan, M., & Tang, Y. (2022). Real-time data-driven dynamic scheduling for flexible job shop with insufficient transportation resources using hybrid deep Q network. Robotics and Computer-Integrated Manufacturing, 74, https://doi.org/10.1016/j.rcim.2021.102283.
Osorio, C., & Selvam, K. K. (2015). Solving Large-scale Urban Transportation Problems by Combining the Use of Multiple Traffic Simulation Models. Transportation Research Procedia, 6, 272–284, https://doi.org/10.1016/j.trpro.2015.03.021.
Qu, Y., & Zhou, X. (2017). Large-scale dynamic transportation network simulation: A space-time-event parallel computing approach. Transportation Research Part C: Emerging Technologies, 75, 1–16, https://doi.org/10.1016/j.trc.2016.12.003.
Rodriguez, S. A., De la Fuente, R. A., & Aguayo, M. M. (2021). A simulation-optimization approach for the facility location and vehicle assignment problem for firefighters using a loosely coupled spatio-temporal arrival process. Computers and Industrial Engineering, 157, https://doi.org/10.1016/j.cie.2021.107242.
Shehadeh, K. S., Wang, H., & Zhang, P. (2021). Fleet sizing and allocation for on-demand last-mile transportation systems. Transportation Research Part C: Emerging Technologies, 132, https://doi.org/10.1016/j.trc.2021.103387.
Straub, F., Maier, O., Gohlich, D., & Zou, Y. (2023). Forecasting the spatial and temporal charging demand of fully electrified urban private car transportation based on large-scale traffic simulation. Green Energy and Intelligent Transportation, 2(1), https://doi.org/10.1016/j.geits.2022.100039.
Vargas, V. M., Gutierrez, P. A., Barbero-Gomez, J., & Hervas-Martinez, C. (2023). Soft labelling based on triangular distributions for ordinal classification. Information Fusion, 93, 258–267, https://doi.org/10.1016/j.inffus.2023.01.003.
Zang, Z., Xu, X., Qu, K., Chen, R., & Chen, A. (2022). Travel time reliability in transportation networks: A review of methodological developments. In Transportation Research Part C: Emerging Technologies (Vol. 143). Elsevier Ltd, https://doi.org/10.1016/j.trc.2022.103866.
Zhang, D., Cao, J., Feygin, S., Tang, D., Shen, Z. J., & Pozdnoukhov, A. (2019). Connected population synthesis for transportation simulation. Transportation Research Part C: Emerging Technologies, 103, 1–16, https://doi.org/10.1016/j.trc.2018.12.014.
Zheng, H., Sun, H., Zhu, S., Kang, L., & Wu, J. (2023). Air cargo network planning and scheduling problem with minimum stay time: A matrix-based ALNS heuristic. Transportation Research Part C: Emerging Technologies, 156, https://doi.org/10.1016/j.trc.2023.104307.
Zhou, J., Shi, Y., Liang, G., Peng, C., & Liu, C. (2024). Mixed transportation optimization model for oilfield water injection synergizing pipeline network and trucks. Geoenergy Science and Engineering, 241, https://doi.org/10.1016/j.geoen.2024.213105.
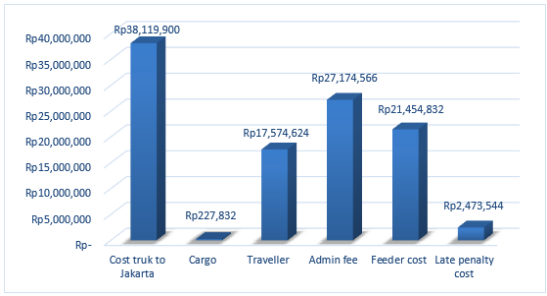
Downloads
Published
How to Cite
Issue
Section
License
Copyright (c) 2024 Farida Sihotang

This work is licensed under a Creative Commons Attribution-ShareAlike 4.0 International License.