Efficiency Evaluation in Indonesia's Quarrying Industry Using Variable Combinations DEA
DOI:
https://doi.org/10.12928/si.v22i2.227Keywords:
Performance evaluation, Quarrying, Variable combinations (VCs), VRS-DEAAbstract
Data Envelopment Analysis (DEA) is a method considered to evaluate a company's performance. DEA applies multiplies the input and output variables for analyzing the efficiency but does not provide guidance in selecting those variables. As a rule, researchers use several methods. If the number of variables used is too many, it will affect the efficiency value. This will reduce the strength of the efficiency value, which can cause all DMU values to be efficient. DEA and variable selection are important in performance evaluation because DEA aids in determining relative efficiency, whereas variable selection guarantees that the evaluation is based on the most relevant and significant aspects. The purpose of this study is to suggest the variable combination method for subtracting the number of variables that will be utilized in implementing the DEA. The method used in this study is the Average Input Variable Combinations (VCs)-Variable Returns-to-Scale (VRS) DEA. The data were classified, defined, and processed with a view to computing efficiency scores and DMU classifications. The research result indicated that the proposed method (VCs-DEA) treats the variable reduction factor and the average calculation factor to obtain the final result of the efficiency score. These two factors contribute to the accuracy of the efficiency value. Some real-world implications of these findings, such as making better use of resources, streamlining operations, and coming up with new plans, Furthermore, the evidence may be used to benchmark performance as well as help decision-makers in creating more effective policy. This study finds that only 1 out of 12 DMUs is efficient (8%), while the remaining 11 are inefficient (92%). Indonesia quarrying establishment can be classified into 3 categories such as Optimal Category (S-Sand); Middle Category (LS-Lime-Stone; F-Feldspars; Gr-Granite; SA-Stone and Andesite; K-Kaolin; Q-Quartz; and G-Gravel); and Less Category (So-Soil; C-Clay; M-Marble; and O-Others).
References
Akhmetova, S. A., & Suleimanova, A. G. (2022). Analyzing the efficiency of green technologies in manufacturing enterprises. Sustainability, 14(2), 947, https://doi.org/10.3390/su14020947.
Ali, M. & Seiford, L. M. (2015). The use of data envelopment analysis in performance measurement: A review of the literature. Journal of the Operational Research Society, 66(3), 355-370, https://doi.org/10.1057/jors.2012.63.
Anouze, A. L. M., & Hamad, I. B. (2019). Data envelopment analysis and data mining to efficiency estimation and evaluation. International Journal of Islamic and Middle Eastern Finance and Management, 12(2), 169-190, https://doi.org/10.1108/IMEFM-11-2017-0302.
Bai, Y., & Sarkis, J. (2017). A data-driven variable selection approach for performance measurement in supply chain management. International Journal of Production Research, 55(10), 2826-2841, https://doi.org/10.1080/00207543.2016.1200312.
Banker, R. D., Charnes, A., & Cooper, W. W. (1984). Some models for estimating technical and scale inefficiencies in data envelopment analysis. Management Science, 30(9), 1078-1092, https://doi.org/10.1287/mnsc.30.9.1078.
Boland L, Légaré F, Perez MM, Menear M, Garvelink MM, McIsaac DI, Painchaud Guérard G, Emond J, Brière N, & Stacey D. (2017). Impact of home care versus alternative locations of care on elder health outcomes: An overview of systematic reviews. BMC Geriatrics, 17(1), 1-20, https://doi.org/10.1186/s12877-016-0395-y.
Brodie, R., & Hsu, C. (2018). Exploring the Relationship Between Mining Policy and Operational Efficiency. Resources Policy, 55, 1-10, https://doi.org/10.1016/j.resourpol.2018.02.008.
Charnes, A., Cooper, W. W., & Rhodes, E. (1978). Measuring the efficiency of decision making units. European Journal of Operational Research, 2(6), 429-444, https://doi.org/10.1016/0377-2217(78)90138-8.
Chen, Z., & Delmas, M.A. (2022). Measuring corporate social responsibility performance in China: A data envelopment analysis approach. Journal of Business Ethics, 180, 713-732, https://doi.org/10.1007/s10551-021-04915-9.
Cooper, W.W., Seiford, L.M., & Zhu, J. (2004). Data Envelopment Analysis. In: Handbook on Data Envelopment Analysis. International Series in Operations Research & Management Science, Springer, Boston, MA., 71, 1-39, https://doi.org/10.1007/1-4020-7798-X_1.
Daneshvar, S. & Adesina, K. (2018a). Integrated data envelopment-thermoexergetic optimization framework for multicomponent distillation system with multiexergetic response in the robust parameter design procedures. Energy Sources, 40, 1491–1507, https://doi.org/10.1080/15567036.2018.1477876.
Daneshvar, S. & Adesina, K. (2018b). Modified variable return to scale back propagation neural network robust parameter optimization procedure for multi-quality processes. Eng. Optim, 50, 1352–1369, https://doi.org/10.1080/0305215X.2018.1524463.
Emrouznejad, A., & Yang, G. L. (2018). A survey and analysis of the state-of-the-art in DEA variable selection. Journal of Productivity Analysis, 49(1), 1-23, https://doi.org/10.1007/s11123-017-0465-3.
García-Sánchez, I.-M., & Rodríguez-Domínguez, L. (2019). Environmental Performance and Economic Efficiency in the Mining Industry: Evidence from the World. Resources, Conservation and Recycling, 148, 1-11, https://doi.org/10.1016/j.resconrec.2019.05.016.
Golany, B., & Roll, Y. (1989). An application procedure for DEA. Omega, 17(3), 237-250, https://doi.org/10.1016/0305-0483(89)90029-7.
Jenkins, L., & Anderson, M. (2003). A multivariate statistical approach to reducing the number of variables in data envelopment analysis. European Journal of Operational Research, 147(1), 51-61, https://doi.org/10.1016/S0377-2217(02)00243-6.
Khan, A. I., & Ghaffar, A. (2023). Reducing input variables in DEA: A novel approach using machine learning techniques. Operations Research Perspectives, 10, 100247, https://doi.org/10.1016/j.orp.2023.100247.
Khezrimotlagh, D., Salleh, S., & Mohsenpour, Z. (2019). A refined data envelopment analysis model for measuring the performance of decision-making units. Computers & Industrial Engineering, 128, 482-489, https://doi.org/10.1016/j.cie.2018.12.058.
Kraidi, A. A., Daneshvar, S., & Adesina, K. A. (2024). Weight-restricted approach on constant returns to scale DEA models: Efficiency of internet banking in Turkey. Heliyon, 10(10), 1-15, https://doi.org/10.1016/j.heliyon.2024.e31008.
Kumar, A., & Singh, P. (2021). Application of DEA with reduced variables: An empirical analysis in the banking sector. International Journal of Financial Studies, 9(4), 45, https://doi.org/10.3390/ijfs9040045.
Liu, W., Zhu, Q., & Shang, J. (2016). A data envelopment analysis model for eco-efficiency evaluation of China's industrial sectors. Journal of Cleaner Production, 142, 2802-2811, https://doi.org/10.1016/j.jclepro.2016.10.154.
Mardani, A., Jusoh, A., & Zavadskas, E. K. (2021). Data envelopment analysis and performance evaluation: A literature review. Operations Research Perspectives, 8, 100167, https://doi.org/10.1016/j.orp.2021.100167.
Mohammad, A., & Yusof, M. (2020). A hybrid DEA approach for variable reduction and performance evaluation. Journal of the Operational Research Society, 71(6), 1023-1035, https://doi.org/10.1080/01605682.2019.1672310.
Nalbantian, L., & Toroslu, I. (2015). Assessing the efficiency of mining firms using DEA. Resources Policy, 46, 33-45, https://doi.org/10.1016/j.resourpol.2014.09.003.
Narasimhan, K., & Ramakrishnan, K. (2019). Reducing dimensionality in DEA models: A practical approach. Computational and Mathematical Organization Theory, 25(4), 507-525, https://doi.org/10.1007/s10588-019-09350-1.
Olesen, O. B., & Petersen, N. C. (2016). Stochastic data envelopment analysisi-A review. European Journal of Operation Research, 251(1), pp.2-21, https://doi.org/10.1016/j.ejor.2015.07.058.
Olsen, T. L. & Karp, L. S. (2019). Data envelopment analysis and the role of variables in measuring efficiency: A review. Journal of Productivity Analysis, 51(2), 117-135, https://doi.org/10.1007/s11123-019-00523-5.
Putri, E. P. & Kusoncum, C. (2020, October). Performance evaluation using PCA-CRS input oriented DEA method. A case study of East Java exports in Indonesia to ASEAN countries. In Proceedings of the 2019 International Conference on “Physics, Mechanics of New Materials and Their Applications”. 445-452, SCI055000. Nova Science Publishers, Inc, https://novapublishers.com/shop/proceedings-of-the-2019-international-conference-on-physics-mechanics-of-new-materials-and-their-applications/.
Putri, E. P., Aduldaecha, S., Putra, B. R. S. P., & Agatha Hannabel Avnanta Puteri, A. H. A. (2024). Performance evaluation of Indonesia's large and medium-sized industries using data envelopment analysis method. OPSI: Jurnal Optimasi Sistem Industri, 17(1), 118-134, https://doi.org/10.31315/opsi.v17i1.11785.
Putri, E. P., Arief, Z., & Yuwono, I. (2023, February). Performance evaluation using input-oriented envelopment DEA method: A case study of micro and small industry in Indonesia. In Physics and Mechanics of New Materials and Their Applications, 2021 – 2022. 289-304, SCI055000. Nova Science Publishers, Inc, https://doi.org/10.52305/QLWW2709.
Putri, E. P., Chetchotsak, D., Jani, M. A., & Hastijanti, R. (2017). Performance evaluation using PCA and DEA: A case study of the micro and small manufacturing industries in Indonesia. ASR: CMU Journal of Social Sciences and Humanities, 4(1), 37-56, https://doi.org/10.12982/CMUJASR.2017.0003.
Putri, E. P., Chetchotsak, D., Ruangchoenghum, P., Jani, M. A., & Hastijanti R. (2016). Performance evaluation of large and medium scale manufacturing industry clusters in East Java Province, Indonesia. International Journal of Technology, 7(7), 1117-1127, https://doi.org/10.14716/ijtech.v7i7.5229.
Shao, Q., Yuan, J., Lin, J., Huang, W., Ma, J., & Ding, H. (2021), A SBM-DEA based performance evaluation and optimization for social organizations participating in community and home-based elderly care services. PloS ONE, 16(3), 1-25, https://doi.org/10.1371/journal.pone.0248474.
Springer, 2022. Math Review: Sets, Functions, Permutations, Combinations, and Notation, Available Online at: https://link.springer.com/content/pdf/bbm%3A978-1-4612-3988-8%2F1.pdf, Accessed on January 25, 2022
Sullivan, L. J., & Mounsey, R. A. (2010). The Role of Technology and Innovation in the Mining Sector. Journal of Cleaner Production, 18(11), 1186-1195, https://doi.org/10.1016/j.jclepro.2010.01.020.
Tavana, M., Khalili-Damghani, K., & Mina, H. (2020). A new dynamic network DEA model for time-series efficiency measurement using goal-directed benchmarking. Measurement, 165, 108145, http://doi.org/10.1016/j.measurement.2020.108145.
Vittal, B., Nellutla, R., & Reddy, M. K. (2021). Selection and analysis of input-output variables using data envelopment analysis of decision making units - Indian private sector banks. International Journal of Engineering and Advanced Technology, 10(5), 119-127, https://doi.org/10.35940/ijeat.E2674.0610521.
Wagner, J.M., & Shimshak, D. G. (2007). Stepwise selection of variables in data envelopment analysis: Procedures and managerial perspectives. European Journal of Operational Research, 180(1), 57-67, https://doi.org/10.1016/j.ejor.2006.02.048.
Wang, J., & Wu, S. (2017). Variable selection for DEA models using a data-driven approach. International Journal of Production Economics, 193, 48-56, https://doi.org/10.1016/j.ijpe.2017.07.007.
Yusoff, N. A., & Chedid, R. (2023). The relationship between innovation, efficiency, and firm performance in the Malaysian SMEs. Journal of Small Business Management, 61(1), 105-123, https://doi.org/10.1080/00472778.2021.1978420.
Zhang, Y., & Wang, Y. (2022). Variable reduction techniques in DEA: A comparative study of factor analysis and principal component analysis. Journal of Productivity Analysis, 58(3), 239-255, https://doi.org/10.1007/s11123-022-00780-9.
Zhu, H., & Zhou, Y. (2021). Measuring operational efficiency and its influencing factors in the logistics industry: Evidence from China. Journal of Transport Geography, 90, https://doi.org/10.1016/j.jtrangeo.2020.102897.
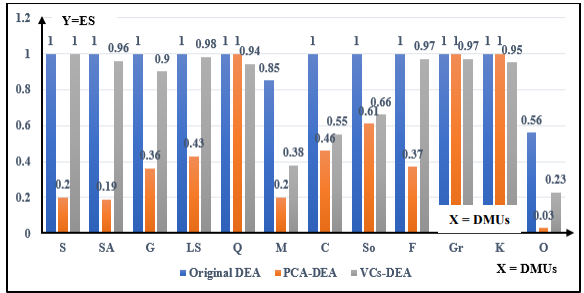
Downloads
Published
How to Cite
Issue
Section
License
Copyright (c) 2024 Erni Puspanantasari

This work is licensed under a Creative Commons Attribution-ShareAlike 4.0 International License.