Sentiment Analysis and Emotional Language as Predictors of Drug Satisfaction in User Reviews
DOI:
https://doi.org/10.12928/si.v22i2.275Keywords:
Sentiment analysis, Healthcare, Emotion detection, Patient satisfaction, PharmaceuticalAbstract
This study investigates how emotional expressions in user-generated drug reviews predict satisfaction ratings using sentiment analysis and emotion detection. By analyzing over 370,000 reviews from the UCI Machine Learning Repository, the study aims to bridge gaps in understanding the emotional drivers behind user satisfaction across different drug categories. For sentiment analysis, VADER, a Python-based lexicon tool, was used to categorize sentiment polarity, while the NRC Word-Emotion Lexicon provided a nuanced mapping of emotions like joy, sadness, and anger. Results reveal that emotions such as joy and trust are positively correlated with higher ratings, while anger and disgust are linked to lower satisfaction. However, the R-squared value (~0.043) indicates that emotions alone do not fully predict ratings, highlighting the need to consider additional factors like drug efficacy and side effects. This low R-squared value suggests that while emotions significantly influence satisfaction, other elements play a substantial role. The study's findings have critical implications for pharmaceutical companies and healthcare providers, suggesting the need for emotion-driven marketing strategies and improved patient support systems. Future research could explore more advanced machine learning models, such as BERT or GPT-based approaches, and investigate specific user demographics or drug side effects to enhance predictive accuracy.
References
Admojo, F. T., Risnanto, S., Windiawati, A. W., Innuddin, M., & Mualfah, D. (2024). Comparison of naive bayes and random forest algorithm in Webtoon application sentiment analysis. Innovation in Research of Informatics (INNOVATICS), 6(1), https://doi.org/10.37058/innovatics.v6i1.10636.
Arifka, D., Hakim, M. N., Adhipta, A. S., Yogananda, K. S. S., Salsabila, R., & Ferdiana, R. (2022). Pandemic fatigue: An analysis of Twitter users’ sentiments against the COVID-19 in Indonesia. Jurnal Psikologi, 49(2), 182–182, https://doi.org/10.22146/jpsi.71979.
Blake, H., Bermingham, F., Johnson, G., & Tabner, A. (2020). Mitigating the psychological impact of COVID-19 on healthcare workers: A digital learning package. International Journal of Environmental Research and Public Health, 17(9), 2997, https://www.mdpi.com/1660-4601/17/9/2997.
Budak, I., Kilic, G., & Organ, A. (2023). Evaluating user experiences of alzheimer’s drugs from online reviews using text mining and sentiment analysis. Uluslararasi Yonetim Bilisim Sistemleri ve Bilgisayar Bilimleri Dergisi, 7(2), 157–167, https://doi.org/10.33461/uybisbbd.1362821.
Chalkias, I., Tzafilkou, K., Karapiperis, D., & Tjortjis, C. (2023). Learning analytics on YouTube educational videos: Exploring sentiment analysis methods and topic clustering. Electronics, 12(18), 3949–3949, https://doi.org/10.3390/electronics12183949.
Czarnek, G., & Stillwell, D. (2022). Two is better than one: Using a single emotion lexicon can lead to unreliable conclusions. PLOS ONE, 17(10), e0275910, https://doi.org/10.1371/journal.pone.0275910.
Evans, J. M., Gilbert, J. E., Bacola, J., Hagens, V., Simanovski, V., Holm, P., Harvey, R., Blake, P. G., & Matheson, G. (2021). What do end-users want to know about managing the performance of healthcare delivery systems? Co-designing a context-specific and practice-relevant research agenda. Health Research Policy and Systems, 19(1), https://doi.org/10.1186/s12961-021-00779-x.
Fang, F., Zhou, Y., Ying, S., & Li, Z. (2023). A study of the ping an health app based on user reviews with sentiment analysis. International Journal of Environmental Research and Public Health, 20(2), 1591, https://doi.org/10.3390/ijerph20021591.
Feng, Y., Yin, Y., Wang, D., Dhamotharan, L., Ignatius, J., & Kumar, A. (2022). Diabetic patient review helpfulness: Unpacking online drug treatment reviews by text analytics and design science approach. Annals of Operations Research, 328(1), 387–418, https://doi.org/10.1007/s10479-022-05121-4.
George, O. A., & Ramos, Q. (2024). Sentiment analysis applied to tourism: Exploring tourist-generated content in the case of a wellness tourism destination. International Journal of Spa and Wellness, 1–23, https://doi.org/10.1080/24721735.2024.2352979.
Golondrino, G. E. C., Alarcón, M. A. O., & Martínez, L. M. S. (2023). Determination of the satisfaction attribute in usability tests using sentiment analysis and fuzzy logic. International Journal of Computers Communications & Control, 18(3), https://doi.org/10.15837/ijccc.2023.3.4901.
Graber, F., Kallumadi, S., Malberg, H., & Zaunseder, S. (2018, April 23). Aspect-based sentiment analysis of drug reviews applying cross-domain and cross-data learning. Proceedings of the 2018 International Conference on Digital Health, https://doi.org/10.1145/3194658.3194677.
Herrera-Peco, I., Jimenez-Gomez, B., Deudero, J. J. P., Gracia, E. B. D., & Ruiz-Nunez, C. (2021). Healthcare professionals’ role in social media public health campaigns: Analysis of Spanish pro vaccination campaign on Twitter. Healthcare, 9(6), 662, https://doi.org/10.3390/healthcare9060662.
Hill, G., Manuell, A., & Willemsen, A. (2023). A rapid review of quality of health information on TikTok. The Journal of Health Design, 8(2), 560–574, https://doi.org/10.21853/jhd.2023.202.
Kimani, J., Karanjah, A., & Kihara, P. (2024). Sentiment classification of safaricom PLC social media sentiments on X (Formerly Twitter). Asian Journal of Probability and Statistics, 26(6), 31–40, https://doi.org/10.9734/ajpas/2024/v26i6622.
Kumaresan, C., & Thangaraju, P. (2023). Sentiment analysis in multiple languages: A review of current approaches and challenges. REST Journal on Data Analytics and Artificial Intelligence, 2(1), 8–15, https://doi.org/10.46632/jdaai/2/1/2.
Lee, H., Lee, S. H., Nan, D., & Kim, J. H. (2022). Predicting user satisfaction of mobile healthcare services using machine learning. Journal of Organizational and End User Computing, 34(6), https://doi.org/10.4018/joeuc.300766.
Li, S., Zhu, B., Zhang, Y., Liu, F., & Yu, Z. (2024). A two-stage nonlinear user satisfaction decision model based on online review mining: Considering non-compensatory and compensatory stages. Journal of Theoretical and Applied Electronic Commerce Research, 19(1), 272–296, https://doi.org/10.3390/jtaer19010015.
Mawadati, A., Ustyannie, W., Wibowo, A. H., & Simanjuntak, R. A. (2024). Analysis of Yogyakarta coffee shop visitor reviews to increase customer satisfaction using sentiment analysis. KnE Social Sciences, https://doi.org/10.18502/kss.v9i10.15693.
Maysyaroh, S., & Rusydiana, A. S. (2023). Twitter sentiment analysis on halal cosmetics. Islamic Marketing Review, 2(1), https://doi.org/10.58968/imr.v2i1.232.
Mittal, D., & Agrawal, S. R. (2022). Determining banking service attributes from online reviews: text mining and sentiment analysis. International Journal of Bank Marketing, 40(3), 558–577, https://doi.org/10.1108/ijbm-08-2021-0380.
Mohammad, S. M. (2009). NRC emotion lexicon. Saifmohammad.com. https://saifmohammad.com/WebPages/NRC-Emotion-Lexicon.htm.
Mohammad, S., Bravo-Marquez, F., Salameh, M., & Kiritchenko, S. (2018, June 1). SemEval-2018 Task 1: Affect in Tweets. ACLWeb; Association for Computational Linguistics, https://doi.org/10.18653/v1/S18-1001.
Rao, S. A., Ravi, M. S., Zhao, J. W., Sturgeon, C., & Bilimoria, K. Y. (2020). Social media responses to elective surgery cancellations in the wake of COVID-19. Annals of Surgery, 272(3), https://doi.org/10.1097/sla.0000000000004106.
Salim, S., Iqbal, Z., & Iqbal, J. (2021). Emotion classification through product consumer reviews. Pakistan Journal of Engineering and Technology, 4(4), 35–40, https://doi.org/10.51846/vol4iss4pp35-40.
Serrano-Guerrero, J., Alshouha, B., Romero, F. P., & Olivas, J. A. (2022). Affective knowledge-enhanced emotion detection in Arabic language: A comparative study. JUCS - Journal of Universal Computer Science, 28(7), 733–757, https://doi.org/10.3897/jucs.72590.
Shao, H., Zhu, M., & Zhai, S. (2023). Mental health diagnosis in the digital age: Harnessing sentiment analysis on social media platforms upon ultra-sparse feature content. https://arxiv.org/pdf/2311.05075.
Shyni, K. V. K. (2022). User generated contents in digital media – A study on customer perception. International Journal of Current Science Research and Review, 05(03), https://doi.org/10.47191/ijcsrr/v5-i3-10.
Yu, X., Wang, H., & Chen, Z. (2024). The role of user-generated content in the sustainable development of online healthcare communities: Exploring the moderating influence of signals. Sustainability, 16(9), 3739, https://doi.org/10.3390/su16093739.
Zhai, Y., Song, X., Chen, Y., & Lu, W. (2022). A study of mobile medical app user satisfaction incorporating theme analysis and review sentiment tendencies. International Journal of Environmental Research and Public Health, 19(12), https://doi.org/10.3390/ijerph19127466.
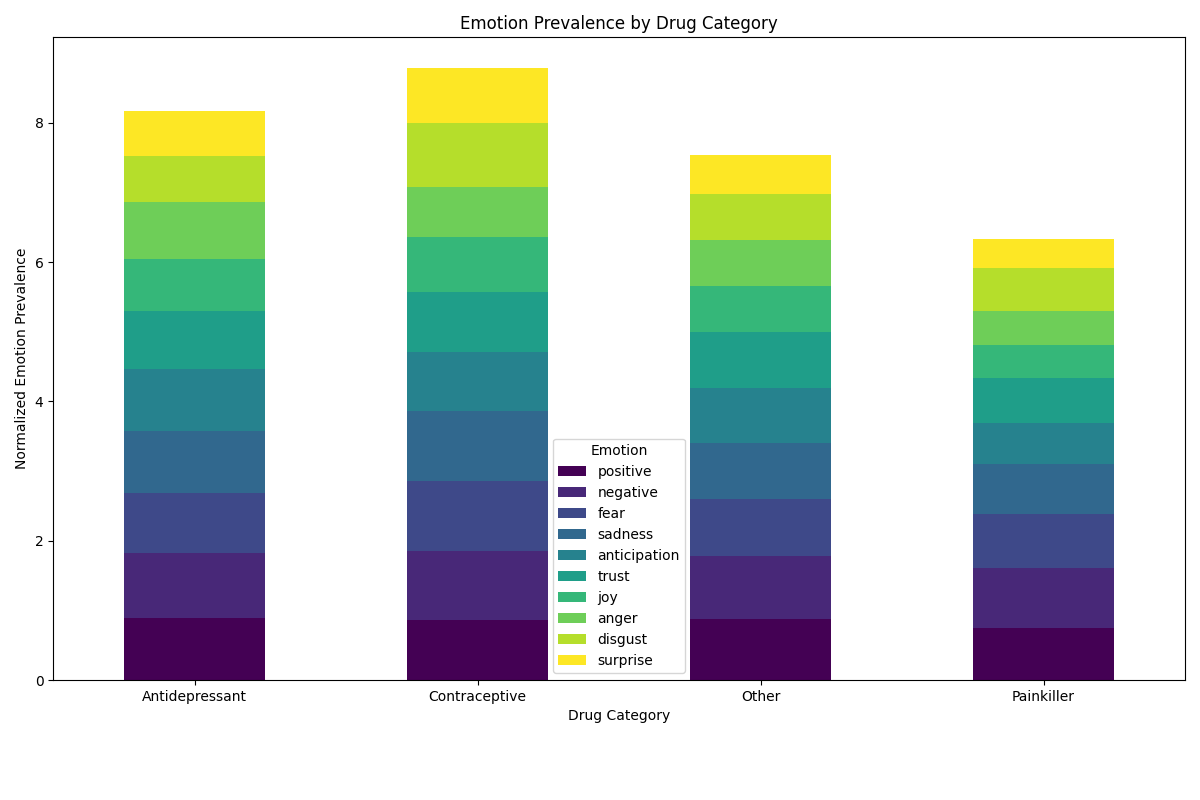
Downloads
Published
How to Cite
Issue
Section
License
Copyright (c) 2024 Ida Lumintu

This work is licensed under a Creative Commons Attribution-ShareAlike 4.0 International License.